A Practical Path to AI Agentic Systems (Part I)
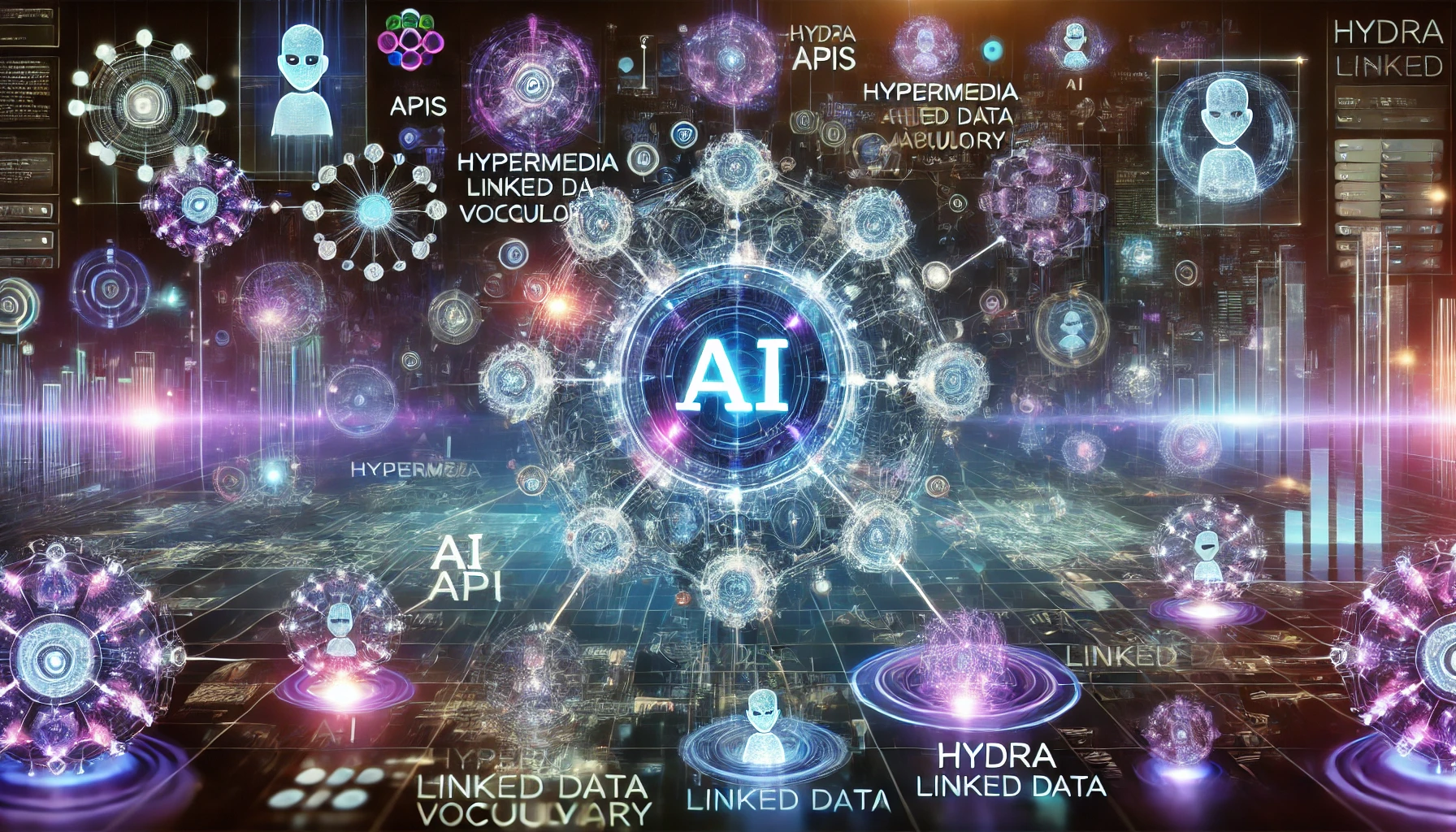
I’ve been designing and building AI-powered applications, on and off, for roughly 15 years. Although I was fortunate to have access to GPT3 about a year ahead of the mainstream, I was wholly unprepared for the wide-reaching impact of this technology. The introduction of ChatGPT in late 2022 brought into sharp focus just how impressive the current generation of large language models have become. The sheer breadth of zero-shot capabilities demonstrated by single model has everyone’s minds racing. There is obviously immense power here, but how do we harness it to realize its true value and potential? That is the trillion dollar question.
One of the most compelling visions for humanity’s next steps with AI is creating autonomous agents; AI systems that can do more than summarize text and provide plausible, information-shaped responses to our prompts. These are AI systems that can interact with other systems or the physical world. Although a great deal of R&D is underway as I write this, I’m shocked that one of the most promising solutions to the conundrum of how to achieve such agentic systems continues to fly under the radar…
Lateral Thinking with Withered Technology
I suspect the primary reason the approach I intend to introduce in this article series isn’t already being talked about is because the ideas aren’t new. In a world plagued with novelty bias and an obsessive pursuit of the next shiny-object we rob ourselves of both the opportunities presented by learning lessons from the past and the “lateral thinking with withered technology.”
“The genius behind this concept is that for product development, you’re better off picking a cheap-o technology (‘withered’) and using it in a new way (‘lateral’) rather than going for the predictable, cutting-edge next-step…
Most product concepts are the result of iterative evolution. They tend to be slightly thinner, faster or differently hued than their competition. You can see them coming a generation away. By contrast lateral products are bold and surprising because they tackle their category orthogonally.”
-Untamed Adam - Nintendo’s Little-Known Product Philosophy
Between 1997 and 2013, we completely solved many of the problems we currently face with agentic systems but the solution did not offer meaningful value in the context of its time. Consequently it was ignored and subsequently forgotten.
“Nothing is more powerful than an idea whose time has come.”
-Victor Hugo
From Conversational Interfaces to AI Agents
My first introduction to natural language, conversational interfaces came in the mid 1990s. I was bored in my “Design/Technology” GCSE class. Consequently, while everyone occupied themselves with tedious busywork, I mostly played with the Acorn RISC PCs dotted along the perimeter of the classroom. Instead of focusing on my coursework I built ELIZA.
ELIZA is a very simple chatbot created to explore communication between humans and machines. It operated on rudimentary pattern-matching and substitution methodologies to create the illusion of understanding. Most commonly it would simply reflect back a rephrasing of inputs which led to the most popular ELIZA script, a Rogerian psychotherapist which mostly transformed statements into questions.
When I showed the results to my classmates, they were both impressed and quickly taken by the ELIZA Effect. I, in contrast, was unsatisfied.
My work moved on to developing my ELIZA into a general purpose chatbot that could carry on a much wider variety of conversations. I spent the next year working on this project to the dereliction of my coursework. Eventually my coursework was due (and representing 60% of my final grade) so I scrambled to compress two years of coursework into two weeks. Somehow I managed to eek out a B and I kept playing with my chatbot.
Around the same time, I was regularly watching Star Trek TNG and I was always impressed with how crew members could talk with the ship’s computer and it would not only talk back, it would perform the tasks they asked! I began to imagine upgrading my chatbot to perform tasks based on my natural language prompts.
Despite a great deal of work, my chat-powered agent could only perform tasks that I had pre-programmed. Moreover I could never truly enable my agent to take actions based on a broad-enough syntax to be practical. All I had accomplished was the creation of a new “fuzzy” syntax for basic system commands. Ultimately I had neither the knowledge nor the computational horsepower to build such a system, but I never forgot that dream.
Fast-forward a quarter-century or so, and the encoder-decoder and transformer architectures allow radically improved prompt inference. The models can now “understand” (in a sense) what we are asking. Perhaps we are entering the age of AI agents.
The Rise (and fall) of the Rabbit R1
Following the AI gold rush precipitated by the release of ChatGPT, we were introduced to a number of agentic AI devices, including the Rabbit R1.
The Rabbit R1 was a device that promised to augment the chat capabilities of these models with their supposedly revolutionary Large Action Model (LAM). I wondered if this would make my silly sci-fi fantasies from the 1990s a reality, but as I read scathing review after scathing review, I quickly realized this was just a fancy GPT wrapper around the same basic system I built last century. In other words, despite improved natural-language inference, it could only execute a limited number pre-programmed tasks.
Investigation into this LAM revealed a handful of playwrite scripts designed to automate the browser in a very rigid way. This explained the limited number of “actions” and why the actions were so brittle.
Now, since the disastrous release of the R1, I’m told the product has gotten better (and we’re seeing progress from different software and hardware vendors). That said, the iterative evolution of the cutting edge is still fraught with problems.
The Limitations of LLMs
Although LLMs demonstrate impressive and clever tricks, they are deeply flawed.
The first problem is that of hallucination. I once asked ChatGPT to generate some code for me and it promptly returned a copy-and-paste ready code snippet for me. This was fantastic… except the code was invalid; the crux of the code was a call to a method that didn’t exist. You see, language models have no concept or understanding of the programming language (or any language). Instead, they rely on patterns observed in the training data. When a response is generated the model iteratively looks for the next probable token based on the prompt and the output produced so far. In my case, the model hallucinated a statistically probable method. The model is not “thinking” and is not “understanding” anything. It’s all just spicy autocomplete.
We’ve managed to paper over the problems of hallucination through techniques such as Retrieval-Augmented Generation (RAG) where we perform more traditional information-retrieval processes to build a concrete context for a model to work from.
Give the AI Chrome?
This style of approach is driving at least one current iterative evolution approach towards agentic systems. Essentially this approach asks “What if we just give the LLM a web browser and have it navigate a UI and figure out how to operate the app?”
There are a few problems to this. First many web apps are hostile to bots and introduce some measures to restrict use to humans. Second, between developer apathy towards accessibility and overcomplicated web development frameworks, it can be difficult for an AI-driven browser to function reliably. There is also the danger of prompt injection.
It remains alarmingly easy to highjack a language-model’s train-of-thought by introducing language that fundamentally changes the instructions. The ever-widening context-windows available in the most cutting-edge models only amplify this problem. Although both bespoke and off-the-shelf guardrails exist to attempt to detect prompt injection, we continue to come up with novel approaches for jailbreaking constrained models to behave in ways they are not supposed to.
What would happen if a bad actor injected a sufficiently well-crafted prompt into the page content? Could that enable the actor to hijack the agent in dangerous ways? Probably, and it’s worth being concerned about.
We also have to contend with the fact that an AI only possesses limited contextual understanding of what it is looking at. Recent high-profile faceplants observed in Google’s AI results have advised users to add glue to pizza sauce (because the model has no concept of a shitpost on reddit), to eat small rocks daily (because the model has no concept of a satirical website), and even advising men to iron their scrotum (because the model incorrectly conflated wrinkles in fabric with wrinkles in skin).
Can we trust a model to find and interact with the right site, or could it find a convincing fake site to enter your credentials into? It seems like we’re back to creating pre-defined behaviors. This is especially true as we get to the problem of resource discovery.
Generally for any software system to interact with another software system, there is some amount of out of band information the client must possess. This typically includes URLs, data structures, functionality, validation rules, etc.
Truth be told, an AI driving a web browser (with a limited amount of out-of-band information) could probably navigate a web application and fumble around until it found the correct screen and functionality to invoke. This remains slow and unreliable.
There’s also the issue of data semantics. A model could probably infer a statistically plausible set of semantics for a form, but these remain probabilistic guesses. Semantics are fairly easy for humans to get right, and even easier for language models to get wrong.
What About Giving the Model CURL?
We can sidestep some of the problems inherent to giving a model access to a web ap by instead having it interact directly with the API. Asking a language model to present information as JSON is not uncommon, and this is a useful way to connect language models to classical code. This does, however, significantly increase the amount of out-of-band information necessary to interact with the system, which prevents agents from being truly autonomous. We still need to pre-program behavior, API docs, JSON schemas, semantics, etc. for every application our agent might interact with.
There is also the problem of semantics. Working directly with an API also removes much of the context necessary for a model to naturally infer semantics. Instead it is only working with decontextualized name/value pairs. The English language is astonishingly vague and many words are overloaded. Language models frequently fail to accurately determine the correct semantics with so little context. We also still run the risk of the model hallucinating an API endpoint or a payload simply because it is statistically likely to exist.
Another glaring issue is the way we currently build APIs. The vast majority of JSON APIs offer an inconsistent interface. Often these are a mix of narrow and/or overloaded RPC calls. Paging mechanisms and filtering is often inconsistent, it is not always clear which API endpoints have side effects, which endpoints are idempotent, etc. As things stand, without a lot of custom programming and a whole pipeline of guardrails, direct API access is even riskier than an agent driving a web browser.
Summary of Challenges
- Hallucination
- LLMs generate responses based on statistical probabilities rather than actual understanding, which can lead to fabricated or incorrect outputs (e.g., nonexistent API calls).
- Techniques like Retrieval-Augmented Generation (RAG) help mitigate this but don’t eliminate the issue.
- AI Agentic Systems & Web Navigation Issues
- Granting an LLM a web browser to navigate and operate applications is problematic due to:
- Bot Restrictions – Many web apps block automated access.
- Accessibility & Complexity – Modern web frameworks often lack consistency, making automation unreliable.
- Prompt Injection Risks – Attackers can manipulate AI behavior through subtle or well-crafted prompts, leading to security vulnerabilities.
- Contextual Misinterpretations – LLMs can’t reliably distinguish between credible and satirical or misleading information, leading to bizarre or harmful recommendations.
- Granting an LLM a web browser to navigate and operate applications is problematic due to:
- Interacting with APIs Instead of Browsers
- Direct API interaction removes UI-related challenges but introduces new problems:
- Increased Out-of-Band Information Needs – The AI needs pre-configured API knowledge, undermining true autonomy.
- Loss of Context & Semantic Misinterpretations – API responses lack the rich context of a UI, making it harder for LLMs to infer meaning accurately.
- Hallucinated APIs & Payloads – The model may fabricate plausible but incorrect API calls, leading to unreliable interactions.
- Direct API interaction removes UI-related challenges but introduces new problems:
LLMs are powerful but deeply flawed, particularly in agentic applications. While strategies like RAG and direct API interaction address some issues, fundamental problems of hallucination, contextual understanding, and security risks remain.
The challenges covered thus far are far from exhaustive. We must also contend with the following realities:
1. Lack of Determinism & Reliability
- LLMs do not guarantee consistent outputs for the same input, making them unreliable for mission-critical tasks.
- Responses can change based on subtle variations in prompts, system updates, or even hidden biases in training data.
2. Security Vulnerabilities Beyond Prompt Injection
- Model Extraction Attacks: Attackers can systematically query the model to extract proprietary knowledge or reproduce its behavior.
- Data Leakage: If trained on sensitive data, an LLM may unintentionally expose private or proprietary information.
- Model Bias Exploits: Bad actors can manipulate AI responses by exploiting known biases or weaknesses in the training data.
3. Limited Real-World Awareness & Adaptability
- LLMs lack real-time learning and adaptation; they rely on pre-trained knowledge and retrieval-based augmentation.
- They struggle with evolving contexts (e.g., breaking news, legislative changes, or dynamic business rules).
4. Computational & Latency Concerns
- Running LLMs at scale requires significant computational resources, increasing cost and environmental impact.
- Real-time interaction, particularly for agentic systems, is often too slow for practical applications.
5. Lack of True Understanding or Reasoning
- LLMs operate on pattern recognition rather than true reasoning, limiting their ability to generalize across unfamiliar tasks.
- Complex multi-step reasoning (e.g., scientific deduction, legal analysis) often results in plausible but incorrect answers.
6. API & Web Navigation Fragility
- Even if an AI agent is given API access, changes in API structures or web interfaces can break automated processes.
- LLMs do not inherently understand error handling or edge cases, making automated interactions unreliable.
7. Ethical & Legal Risks
- Potential for misinformation: Incorrect but confident-sounding answers can mislead users in high-stakes domains (e.g., medical, legal, financial).
- Liability concerns: If an AI agent performs an unintended action (e.g., making unauthorized transactions), accountability is unclear.
- Regulatory challenges: Many jurisdictions are still developing legal frameworks for AI-generated content and autonomous decision-making.
While LLMs and agentic systems hold promise, their use in autonomous decision-making and system interaction when following iterative evolution remains risky. Their lack of determinism, security vulnerabilities, and inability to adapt in real-time pose significant challenges that require careful mitigation strategies.
So What’s the Answer?
That, dear reader, will have to wait for part II of this series. We will tackle the major problems one-by-one to pave a pragmatic path towards truly autonomous AI agents.